How does MAGMA work?
MAGMA performs a GSA that shows which gene families are associated with a trait. To achieve this, MAGMA follows these steps:
- Annotation. In this first stage, MAGMA maps variants to genes. That is to say, the tool determines which variants belong to each gene. To do so, you will need a Reference Genome Annotation (in GTF or GFF format) to extract information on the coding regions in the genome. It is also possible to determine a window to enclose variants that are outside a gene but might be related to it (e.g., within the promoter region).
- Gene Analysis. Once it is clear which genes have variants, MAGMA associates genes with traits using different models:
- Mean SNP-wise association. This involves creating a distribution of SNP p-values and then using a sampling distribution to calculate the statistical significance of the association between the gene and the phenotype.
- Top SNP-wise association. Utilizing the top-N SNPs, an empirical gene p-value is obtained through a permutation procedure.
- Multiple Linear Principal Components Regression. While the other two models can be found in other tools for gene-trait associations, this model is exclusive to MAGMA. A Principal Component Analysis (PCA) is obtained using genotype data. Then, it uses a regression model to associate genes and phenotypes.
- Gene Set Analysis (GSA). Two different types of GSA can be performed:
- Competitive GSA. This statistical test evaluates if genes in a gene set are more associated with a phenotype than genes from others.
- Self-contained GSA. It tests whether genes in a gene set are jointly associated with a phenotype, without taking into account genes from other gene sets.
Performing Gene Set Analysis of GWAS data using OmicsBox
Obtain VCF Files with our Variant Calling Tools
As explained in another OmicsBox blog, to obtain VCF files with variant information for association with traits it is necessary to align FASTQ files and then use one of our Variant Calling Tools to generate this type of file. Unreliable variants with low quality can be filtered out without Variant Filtering Tool.
An example of OmicsBox workflow from FASTQ files to GWAS can be seen in Figure 1:
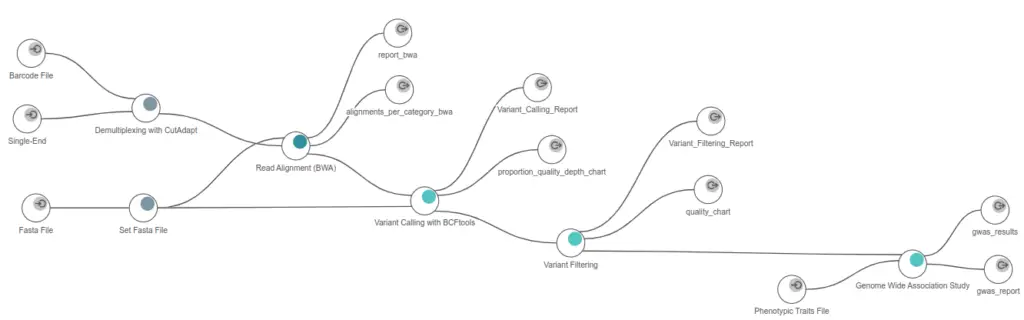
Perform GWAS to Associate Variants and Phenotypes
In another blog, we discussed best practices for conducting GWAS correctly. It is important to filter out variants with too many missing data points in order to increase statistical significance without reducing the number of variants excessively. Additionally, selecting the correct GWAS model is crucial. You can get more information about choosing a suitable model in the User Manual’s GWAS entry.
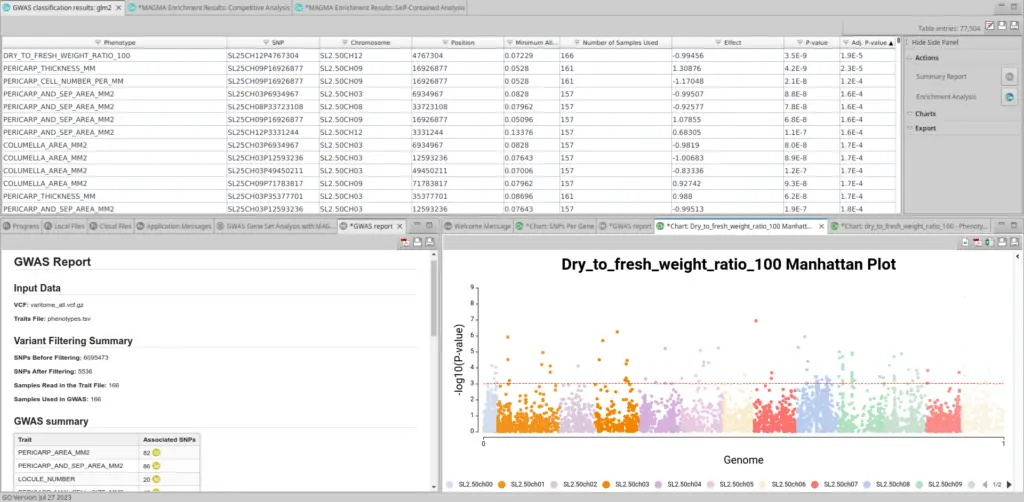
Run Gene Set Analysis with MAGMA
Once you have the GWAS table in OmicsBox, click on Enrichment Analysis in the Actions sidebar. A new wizard will open. On the first page, you can select the phenotype you are interested in and the necessary inputs: the VCF file used in the GWAS step, a Reference Genome Annotation (in GTF or GFF format), and an annotation file containing the genes within each gene set. This annotation file can be easily exported from OmicsBox as a box file or a text file.
On the next page, you will be able to select the window for including SNPs within a gene (in kilobases) and choose various options for the GSA, such as the gene test model to use, how to rank genes in the GSA, and the size limits you want to apply to your gene sets. Additionally, you can opt for the self-contained GSA (see Figures 3 and 4).
Visualization of Results
First of all, in the MAGMA table, you can see which gene sets are significantly associated with the phenotype. In addition, you can access more information about the genes within that gene set and the variants present in these genes (see Figure 5).
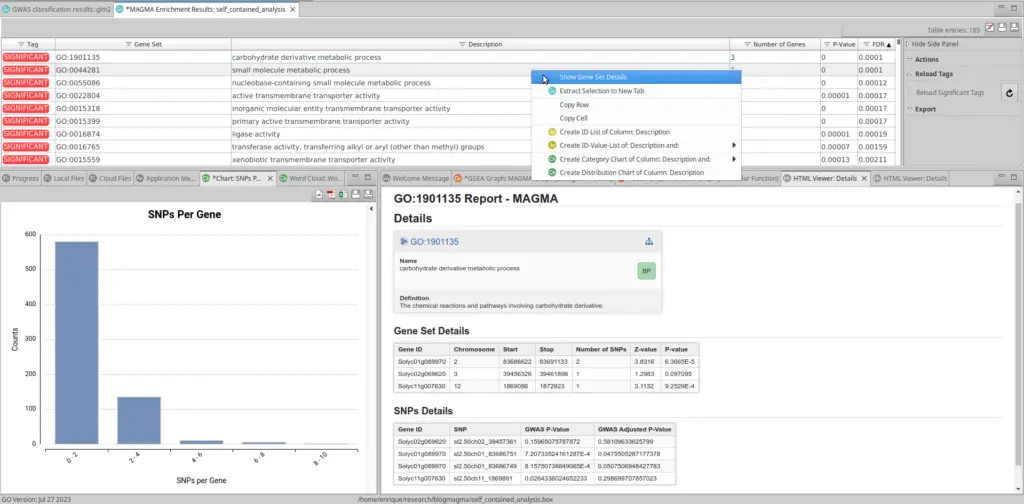
Furthermore, using MAGMA within OmicsBox means that you will be able to access powerful visualization tools. You can analyze informative plots and graphs to visualize the results of GSA. These visuals help convey the biological relevance of identified gene sets and make it easier to interpret the data.
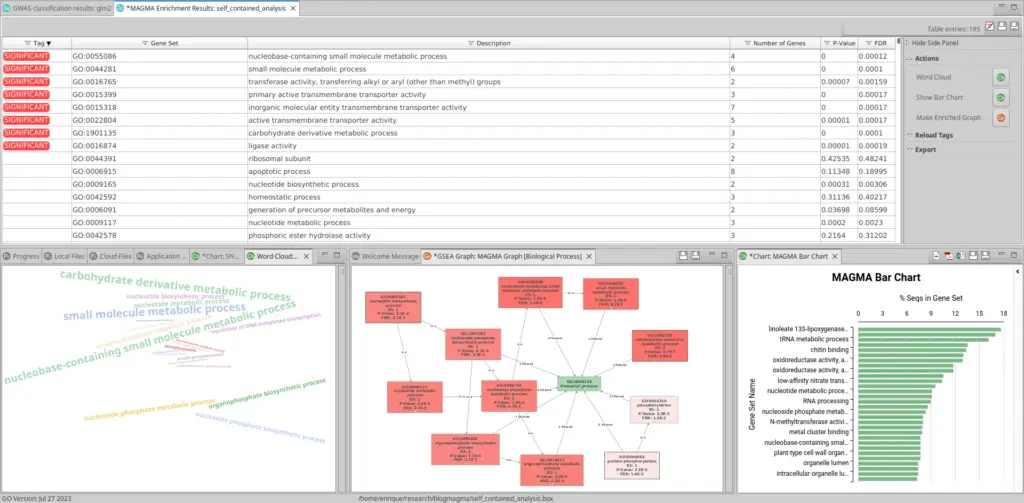
Conclusion
In genetics, understanding the intricate relationships between genes and traits is a complex puzzle. GWAS has assisted us in identifying genetic variants associated with diseases and traits. Still, MAGMA takes us a step further by providing a broader overview of which gene families are linked to a trait. Furthermore, the visualization tools implemented in OmicsBox make this task easier for biologists. So, whether you’re studying the genetic basis of a disease or unraveling the genetics of complex traits, MAGMA proves to be a valuable ally in your research.
In this article, we have explored the power of GSA in the context of GWAS, specifically focusing on how MAGMA can support researchers in this pursuit. With this knowledge, you are better equipped to research the genetic intricacies that shape life.
References
de Leeuw C, Mooij J, Heskes T, Posthuma D (2015): MAGMA: Generalized gene-set analysis of GWAS data. PLoS Comput Biol 11(4): e1004219. doi:10.1371/journal.pcbi.1004219
de Leeuw C, Neale BM, Heskes T, Posthuma D (2016): The statistical properties of gene-set analysis. Nat Rev Genet 17(6): 353-64. doi:10.1038/nrg.2016.29
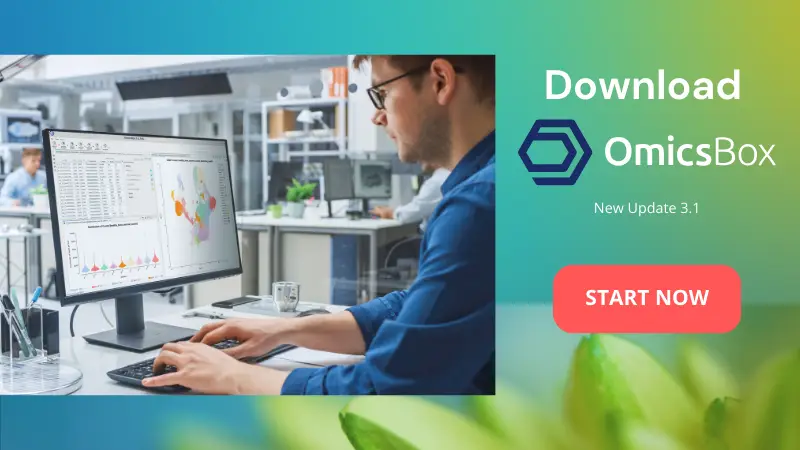
About the Author
Enrique Presa
With a biological and technological academic background, including a BSc in Biotechnology and an MSc in Bioinformatics, Enrique's expertise lies in the areas of Long Reads and Genetic Variation.
The pursuit of genetic advancement in agricultural crops is a nuanced and ongoing scientific endeavor. The advent of Genome-Wide Association Studies (GWAS) marked a significant stride, offering a window into the intricate interplay between genetic variants and phenotypes. Yet, the scope of insights garnered through GWAS can be further enriched when coupled with refined analytical tools. The Gene Set Analysis (GSA) of GWAS data turns out to be a powerful tool that can unveil associations between genetic variants and phenotypes at a gene-set level.
This article aims to elucidate the methodology of employing GWAS and GSA, in particular, MAGMA, in tandem to unravel the genetic underpinnings of phenotypic traits, thereby contributing to the broader narrative of genetic enhancement in agriculture.
How does MAGMA work?
MAGMA performs a GSA that shows which gene families are associated with a trait. To achieve this, MAGMA follows these steps:
- Annotation. In this first stage, MAGMA maps variants to genes. That is to say, the tool determines which variants belong to each gene. To do so, you will need a Reference Genome Annotation (in GTF or GFF format) to extract information on the coding regions in the genome. It is also possible to determine a window to enclose variants that are outside a gene but might be related to it (e.g., within the promoter region).
- Gene Analysis. Once it is clear which genes have variants, MAGMA associates genes with traits using different models:
- Mean SNP-wise association. This involves creating a distribution of SNP p-values and then using a sampling distribution to calculate the statistical significance of the association between the gene and the phenotype.
- Top SNP-wise association. Utilizing the top-N SNPs, an empirical gene p-value is obtained through a permutation procedure.
- Multiple Linear Principal Components Regression. While the other two models can be found in other tools for gene-trait associations, this model is exclusive to MAGMA. A Principal Component Analysis (PCA) is obtained using genotype data. Then, it uses a regression model to associate genes and phenotypes.
- Gene Set Analysis (GSA). Two different types of GSA can be performed:
- Competitive GSA. This statistical test evaluates if genes in a gene set are more associated with a phenotype than genes from others.
- Self-contained GSA. It tests whether genes in a gene set are jointly associated with a phenotype, without taking into account genes from other gene sets.
Performing Gene Set Analysis of GWAS data using OmicsBox
Obtain VCF Files with our Variant Calling Tools
As explained in another OmicsBox blog, to obtain VCF files with variant information for association with traits it is necessary to align FASTQ files and then use one of our Variant Calling Tools to generate this type of file. Unreliable variants with low quality can be filtered out without Variant Filtering Tool.
An example of OmicsBox workflow from FASTQ files to GWAS can be seen in Figure 1:
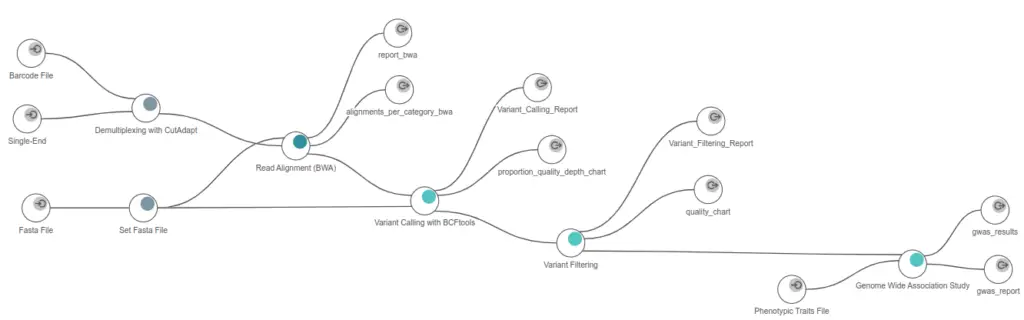
Perform GWAS to Associate Variants and Phenotypes
In another blog, we discussed best practices for conducting GWAS correctly. It is important to filter out variants with too many missing data points in order to increase statistical significance without reducing the number of variants excessively. Additionally, selecting the correct GWAS model is crucial. You can get more information about choosing a suitable model in the User Manual’s GWAS entry.
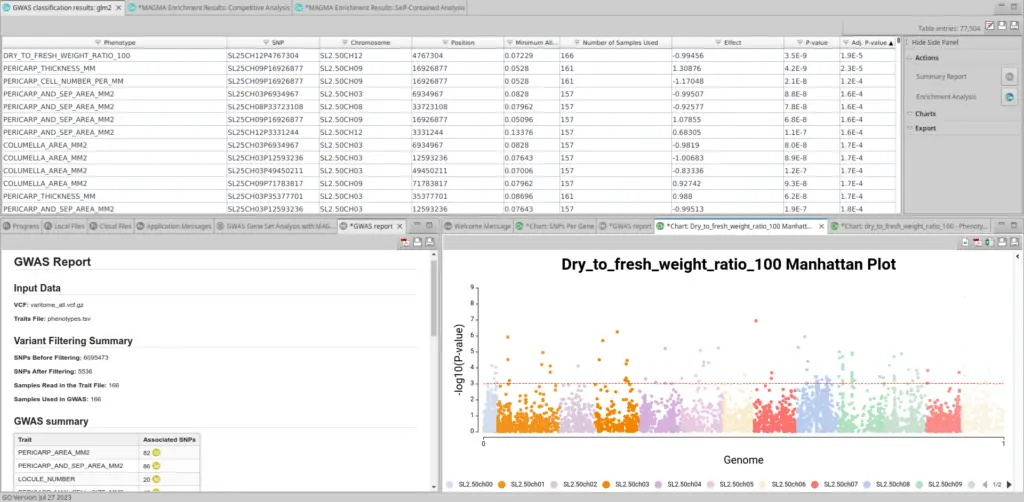
Run Gene Set Analysis with MAGMA
Once you have the GWAS table in OmicsBox, click on Enrichment Analysis in the Actions sidebar. A new wizard will open. On the first page, you can select the phenotype you are interested in and the necessary inputs: the VCF file used in the GWAS step, a Reference Genome Annotation (in GTF or GFF format), and an annotation file containing the genes within each gene set. This annotation file can be easily exported from OmicsBox as a box file or a text file.
On the next page, you will be able to select the window for including SNPs within a gene (in kilobases) and choose various options for the GSA, such as the gene test model to use, how to rank genes in the GSA, and the size limits you want to apply to your gene sets. Additionally, you can opt for the self-contained GSA (see Figures 3 and 4).
Visualization of Results
First of all, in the MAGMA table, you can see which gene sets are significantly associated with the phenotype. In addition, you can access more information about the genes within that gene set and the variants present in these genes (see Figure 5).
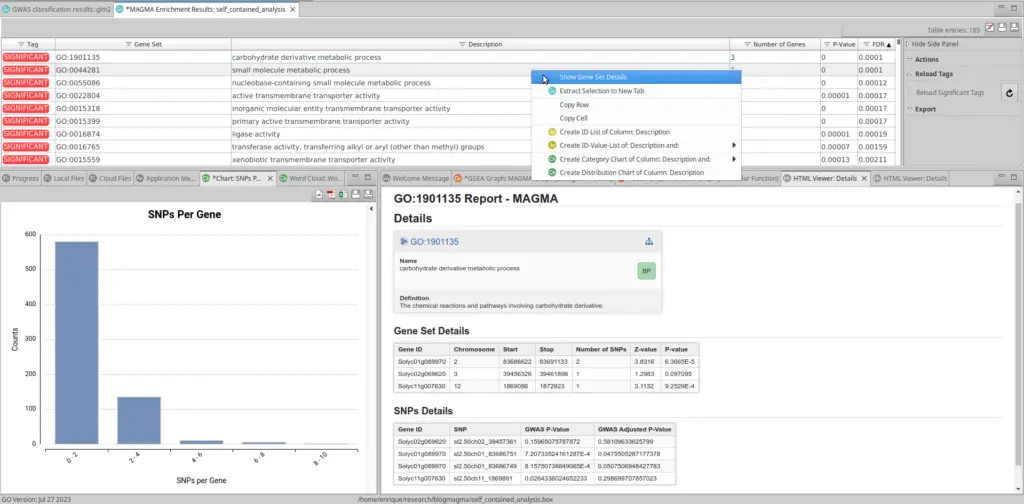
Furthermore, using MAGMA within OmicsBox means that you will be able to access powerful visualization tools. You can analyze informative plots and graphs to visualize the results of GSA. These visuals help convey the biological relevance of identified gene sets and make it easier to interpret the data.
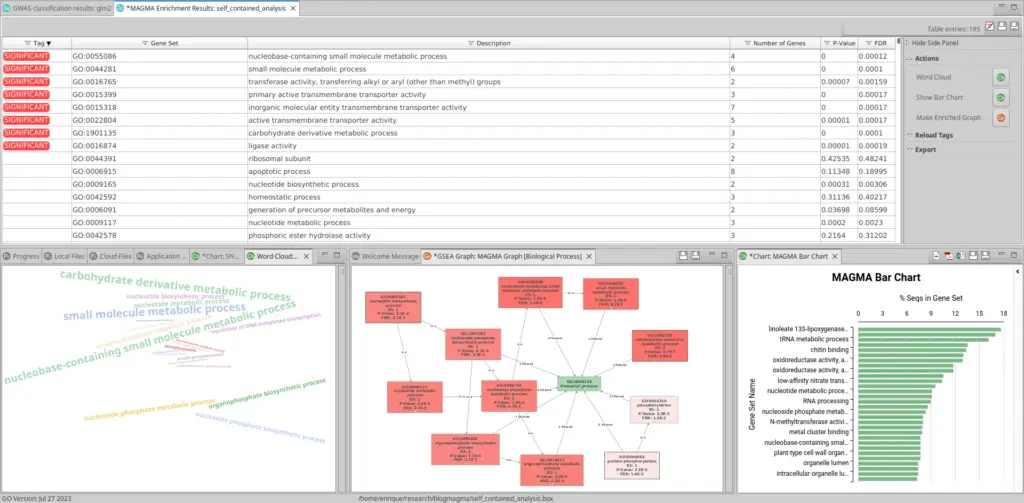
Conclusion
In genetics, understanding the intricate relationships between genes and traits is a complex puzzle. GWAS has assisted us in identifying genetic variants associated with diseases and traits. Still, MAGMA takes us a step further by providing a broader overview of which gene families are linked to a trait. Furthermore, the visualization tools implemented in OmicsBox make this task easier for biologists. So, whether you’re studying the genetic basis of a disease or unraveling the genetics of complex traits, MAGMA proves to be a valuable ally in your research.
In this article, we have explored the power of GSA in the context of GWAS, specifically focusing on how MAGMA can support researchers in this pursuit. With this knowledge, you are better equipped to research the genetic intricacies that shape life.
References
de Leeuw C, Mooij J, Heskes T, Posthuma D (2015): MAGMA: Generalized gene-set analysis of GWAS data. PLoS Comput Biol 11(4): e1004219. doi:10.1371/journal.pcbi.1004219
de Leeuw C, Neale BM, Heskes T, Posthuma D (2016): The statistical properties of gene-set analysis. Nat Rev Genet 17(6): 353-64. doi:10.1038/nrg.2016.29
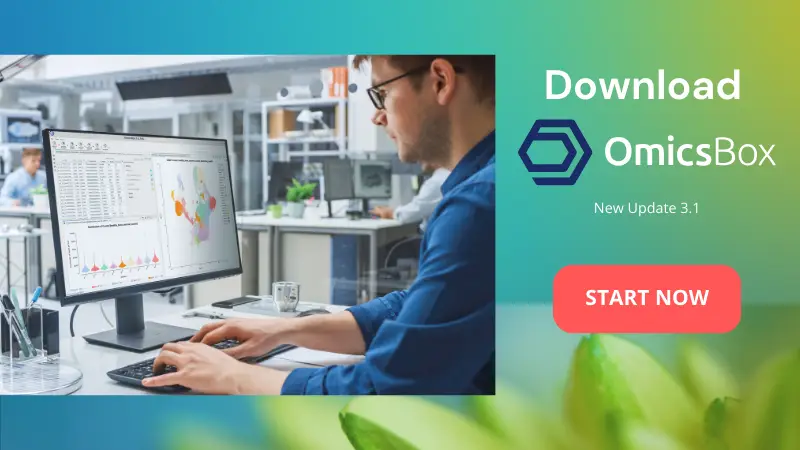
About the Author
Enrique Presa
With a biological and technological academic background, including a BSc in Biotechnology and an MSc in Bioinformatics, Enrique's expertise lies in the areas of Long Reads and Genetic Variation.